Deep Learning-Based Anomaly Detection for Outdoor Home Surveillance
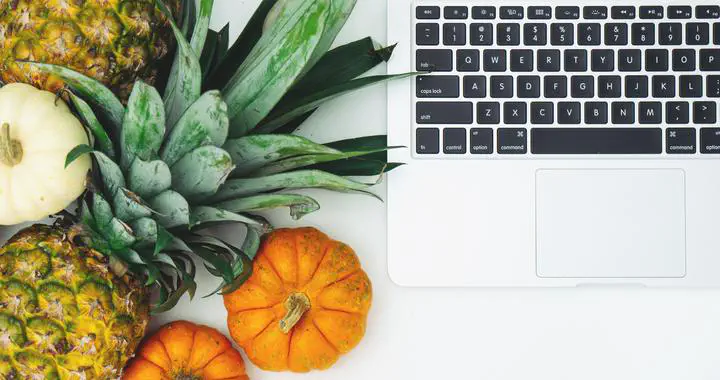
Description
This research project focuses on developing an advanced deep learning (DL) model specifically designed to detect anomalies in outdoor home surveillance systems, addressing the common issue of false alarms caused by non-threatening events. While traditional motion-detecting cameras can trigger alerts for any detected movement, they often result in unnecessary notifications due to factors such as animals, moving vegetation, or changes in lighting. These false alarms create frustration for users, highlighting the need for a more intelligent system that can distinguish between normal and suspicious activities.
To overcome these limitations, we propose a specialized deep learning model tailored for outdoor home surveillance that can accurately detect and classify unusual events. Unlike existing anomaly detection systems designed for public spaces, such as detecting crimes like burglary or vandalism, our model is uniquely focused on the home environment, ensuring it is fine-tuned to recognize typical outdoor scenarios and distinguish real threats from false triggers.